Four CU Boulder aerospace students earn major NASA awards
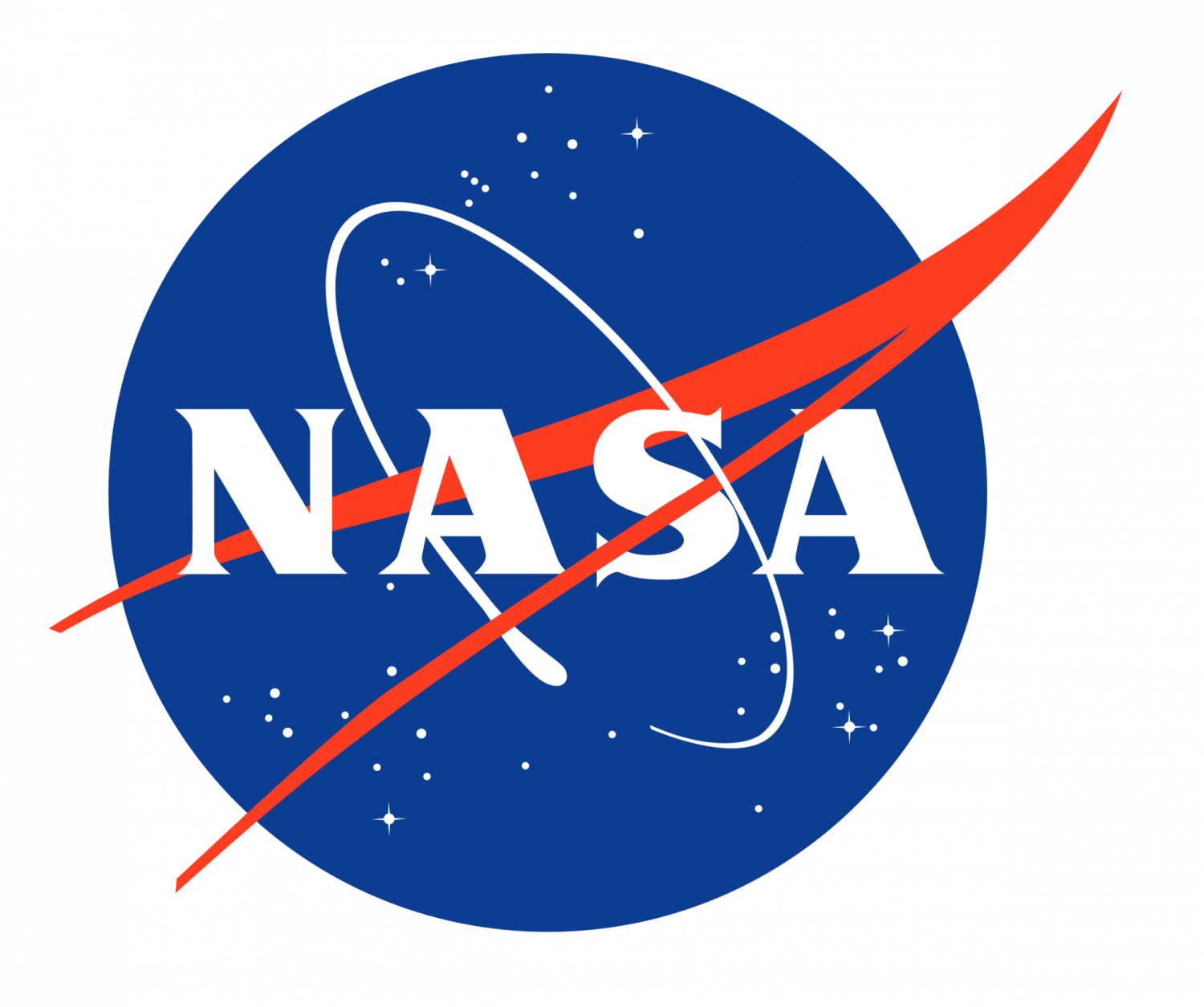
Four University of Colorado Boulder aerospace graduate students have been named 2021 Future Investigators in NASA Earth and Space Science and Technology (FINESST).
Daniel da Silva, Sarah Luettgen, Riley Reid, and Kevin Sacca have each earned the grants, which provide up to $45,000 annually for three years for tuition and to cover graduate student-designed and performed research projects.
FINESST proposals must address goals relevant to NASA's science mission directorate divisions -- heliophysics, earth science, planetary science, or astrophysics. The agency received 835 applications from students across the country for 2021 and is funding 130 of them.
Find out more about each of our awardees and their research below:
Categories: News