Imperfect Match: Short Version
by Ellie Haberl; illustrated by Stuart Sachs
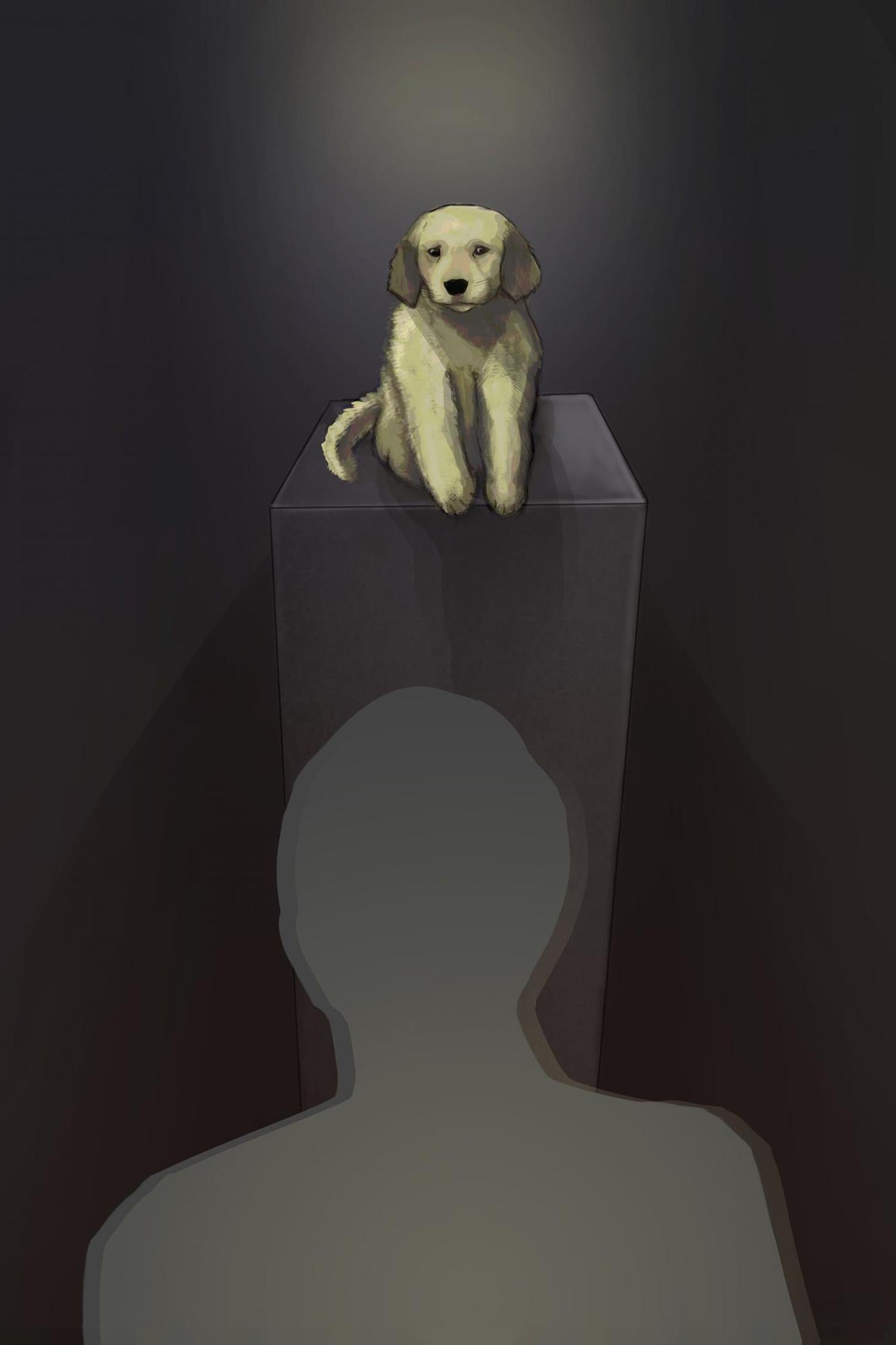
“Ah, here it is! Your match!”
She pasted on a smile, and said, “It looks like you will be taking home Buttercup, a blond-haired golden retriever!.”
But, inside, Lucy was just angry. Lucy had been working at the pet adoption shelter all summer. And she was starting to see a trend. She wondered to herself “Another golden retriever?”
Lucy has loved animals since she was a little girl. She still remembers the day her mom took her to a nearby pet shelter to choose one dog that they would adopt. As soon as she saw Mac, she knew he belonged to her. Mac was love. Now, as a sophomore in high school, she worked at the pet shelter, helping animals get matched with their new owners.
Last December, the owners of the shelter, Rebecca and Mario Thornton, came into the office with a new laptop for Lucy. “Lucy, check out this new computer program we found to help match owners and pets!”
Mario continued, “The team that built the program used machine learning and artificial intelligence (AI) to devise a model that can predict the best fit between an animal and a family. They built the model by taking data from hundreds of pet owners and their reported satisfaction with their pets. This “training data” produced a model that predicts the best pet for each family!
Weeks passed and Lucy enjoyed using the program, but she had started to notice a pattern. Diego, a fellow programmer and her best friend in the robotics club, visited the shelter to bring her ice cream one night, and Lucy shared her observation. “I just have this feeling. Some breeds are getting matched over and over again. It’s weird. And pets with disabilities never, ever get matched. And I have given away more blond golden retrievers than there are blond golden retrievers on the planet!”
Lucy added, “During last week’s robotics club meeting, I started doing a little research on the PerfectMatch model. I noticed that it weights certain variables more than others, like hair color. Don’t you think that’s a terrible metric for pet satisfaction? Plus, the model never matches big dogs with families who live in apartments! Maybe the family lives near a park where the dog can run around and maybe they really want a big dog! The model should be more nuanced and include that information.”
Lucy continued, “I think about the way Mac would be described in the data, before I found him. He barked all the time when I first adopted him, but he actually just needed a better trainer!”
Diego added, “I see what you mean. When I look at the model, I can see it is giving more weight to breed than personality. So, retrievers might be chosen more frequently than dogs that are also easygoing, but maybe belong to a different breed.”
Lucy had brought Mac to work with her that day and he curled up in her lap. It made her so angry to imagine that she wouldn’t have been matched with him. She continued, “And here’s the other thing, Diego. I know fewer people adopt dogs with disabilities in the first place! So maybe there is a smaller amount of data on those dogs in the training data that built the original model. Or maybe the training data relied more heavily on owners who had returned dogs with disabilities. Mac is blind in one eye and I much preferred him to some dogs I know with perfect vision.” Lucy laughed.
Diego joined in, “So there you have it. Mac is the match that makes you happy, but the AI model we’re looking at now probably would have recommended a blonde lab with 20/20 vision because pet owners who reported their experience with pets were already a biased sample.
Lucy wondered, “But Mario said the model is trained from hundreds of families. It should be pretty good, right? Why is this strange thing happening?"
Diego responded, “I guess the sample isn’t large or diverse enough. As just one example, I can see that the training data was primarily taken from suburban neighborhoods where larger houses and larger dogs are more common, so that is probably skewing the recommendations for other families.”
Lucy lifted her spoon “Ok. Here’s my plan. Let’s eat the rest of this ice cream carton, and then write up a case for ditching PerfectMatch that we can present to Mario and Rebecca.
“And maybe offer to build a fairer model by using fairer training data?” Diego asked.
“Yes! Exactly! It’s the bias in the training data that is producing a biased model. We can use a fairer sample for a new model, and make the program open source, so more pets will be matched with more families. Just think of all those animals and people who are waiting to be a family!”
They both smiled.
Final Word Count: 853
Developed by AI Ethics Project 2020. This work is licensed under a Creative Commons Attribution 4.0 International License.
Citation: Haberl, E. (2020). Imperfect Match. V.1.0. Illustrated by S. Sachs. In B.Dalton and T. Yeh (Eds.), AI Stories Series. Available INSERT URL.
Acknowledgement: This work is supported by National Science Foundation Stem+C Award #1934151 to T. Yeh, S. Forsyth and B. Dalton, University of Colorado Boulder.
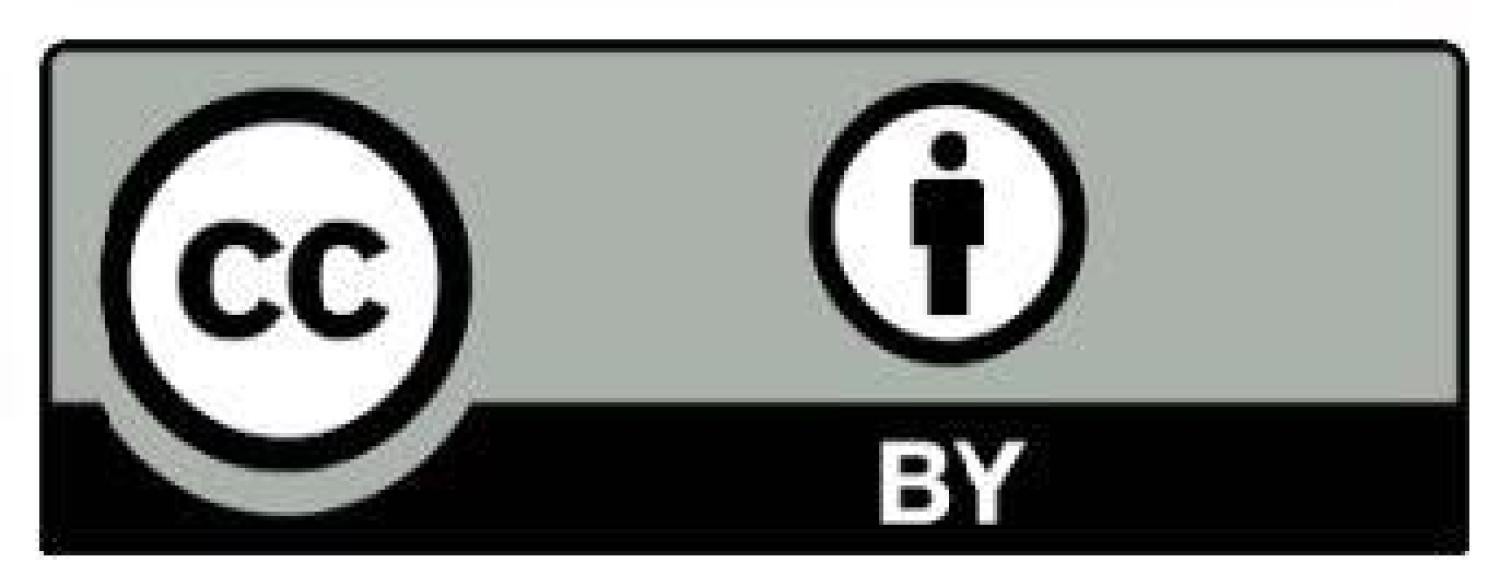